Dylan McKibbon raises the question of how the use of generative AI might impact research ethics and advocates for AI built specifically for medical research in Canada.
__________________________________________
ChatGPT, a controversial Large Language Model (LLM) falling under the wider umbrella of generative AI, is the fastest-growing consumer application in history. Despite how little we understand about the associated risks and extent to which LLMs are used in human activities such as business, government, public and private institutions, academia, matters of law and justice, military bodies, intelligence agencies, political campaigns, financial institutions, philanthropic organizations, insurance agencies, and lobbyists groups, there are no signs of slowing down. Arguably, those who utilize AI could have incredible advantages over those who do not.
Existing literature reveals a recurring pattern: initial excitement about the vast opportunities offered by LLMs tempered by an explication of the associated risks, followed by calls for caution, enhanced regulation, and oversight. Regulation usually falls short of offering a concrete solution to the risks.
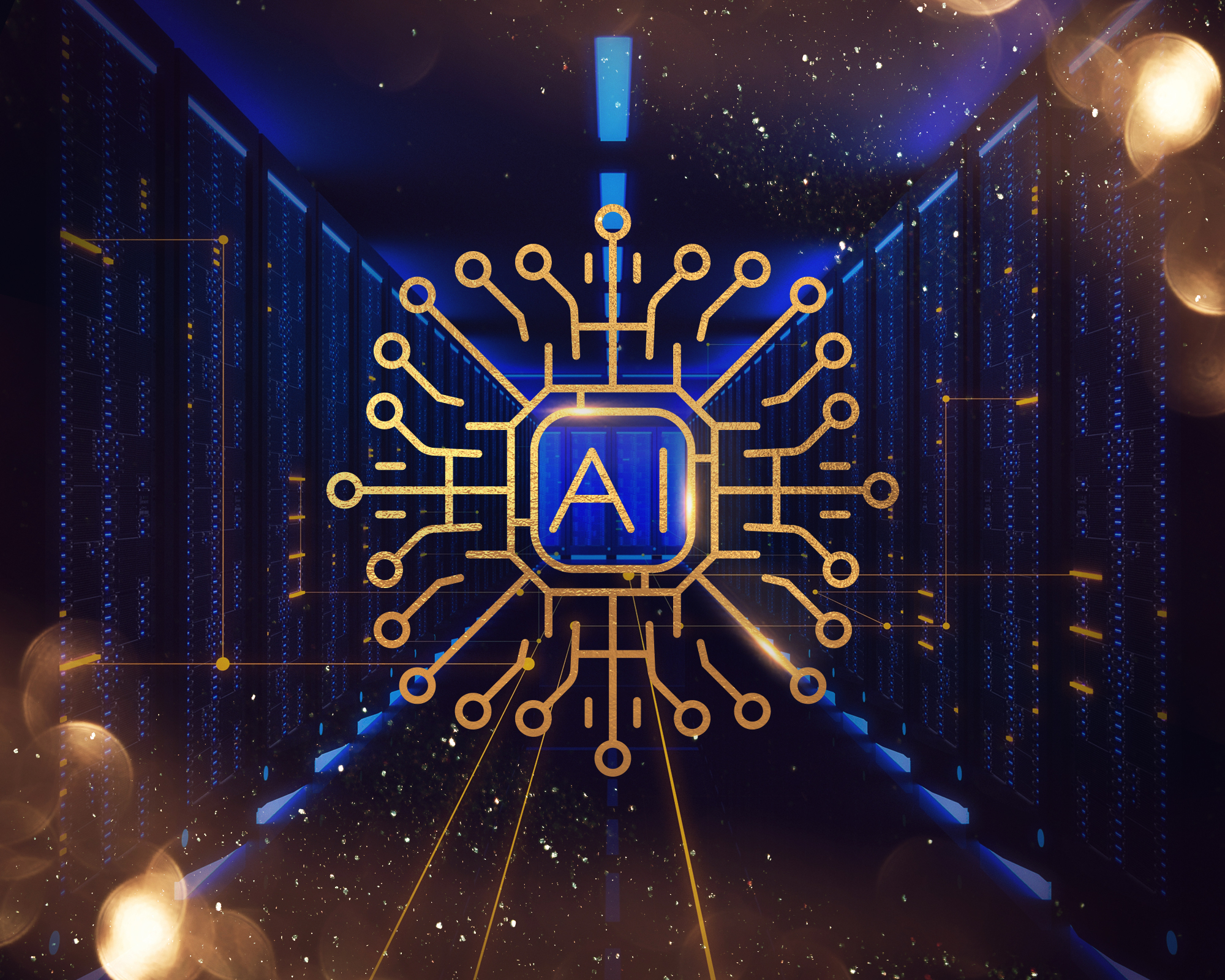
Photo Credit: Creative Commons. Image Description: An illustration symbolizing artificial intelligence (AI), machine learning, and intelligent computing systems.
With deep fakes, language models, image generators, voice emulators, and now video generation, it is increasingly difficult to believe what we see, hear, or read. A wave of distrust has begun crashing against the confidence we once had in what we believe to be “true” and “real”. In this climate of uncertainty an urgent question emerges: how will these perturbations be felt in the field of research ethics? There are already many examples of LLMs being used to generate research-related documents, such as consent forms, research protocols, and grant proposals. Research Ethics Boards (REBs) are not equipped to deal with this rapidly shifting research environment.
Can these AI-generated research documents be identified by REBs? Can they be sufficiently evaluated if REBs do not understand how the LLM works? Is it acceptable for REBs to consult LLMs for their reviews, and if so, can inherent bias be mitigated? If REBs use LLMs for the evaluation of research, can it really be said that ethical perspectives have been thoughtfully considered? Will reliance upon these technologies result in a loss of ethical ability and aptitude? Does the use of LLMs dilute the legal responsibilities of researchers? Could LLMs replace REBs altogether?
These questions are the tip of the iceberg. Researchers don’t really know how LLMs work (most LLMs operate as a kind of “black box”). The identification and evaluation of AI generated research documents will consequently be difficult and ethically problematic. Every LLM evolves in the context of its own unique “ecology”, so each LLM is unique unto itself. We could try to create a “one size fits all” framework for managing the risks presented by the distinctive variations in the ecology of generative AI, but it is likely that our efforts would be quickly outpaced by the evolutionary speed of these technologies.
The best approach may be to create a “path of least resistance” for researchers to incorporate generative AI into their work. This would involve the creation of an independent, Canadian-run, scientifically validated LLM purpose built for research in Canada – one that is non-partisan, subject to oversight, transparent (i.e. Glass Box), and upholds the ethical standards of research, rather than compromising them. LLM capacity has already extended toward the processes of peer review, journal review, finding research sources and citations, analyzing and interpreting literature reviews, interdisciplinary collaboration, and scholarly publishing. Efforts are now underway to lay the groundwork for the creation of flagship datasets for use in behavioral and biomedical research. A language model built specifically for research is a logical step in this progressive evolution.
It can’t just be any mediocre research-focused language model either – it must be the best. It must be the gold standard for research-focused language models in Canada. There are many possible benefits to this approach. A Canadian-specific research ethics LLM that adheres to Canadian regulations and ethical standards could allow researchers to focus more on the substantive aspects of their work rather than the administratively burdensome preparation of research ethics documents.
Canada’s research landscape is subject to regulatory changes and is composed of diverse regions with unique research priorities and challenges. A Canadian-specific LLM could be quickly updated and adapted to incorporate new regulations, guidelines, and domain-specific knowledge that is contextually relevant to the research landscape. Perhaps it could be customized to address regional differences, providing tailored support to research centres across the country enabling greater understanding and adaptation to the linguistic and cultural diversity of Canada.
Ultimately, the widespread use of LLMs will generate profound changes in the organization of human activity. In a sense, AI is seizing control of the ship by becoming the sea. We’ll fail in attempts to responsibly steward the evolution of AI in Canadian research if we rely only upon governance and regulatory frameworks, which are insufficient at best, and deeply harmful at worst. When it comes to AI in research, there are two options: become the sea or drown in it.
__________________________________________
Dylan McKibbon is a Health Privacy Officer with a background in Philosophy and Research Ethics. He is also a member of the Health Sciences North Research Ethics Board, and a Clinical Ethics Intern in the Ethics Quality Improvement Lab at William Osler Health System.